Coupling Process-Based Models and Machine Learning Algorithms for Predicting Yield and Evapotranspiration of Maize in Arid Environments
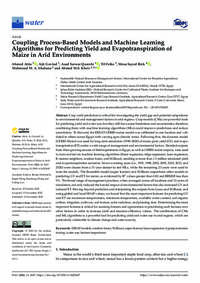
Authors:
Crop yield prediction is critical for investigating the yield gap and potential adaptations to environmental and management factors in arid regions. Crop models (CMs) are powerful tools for predicting yield and water use, but they still have some limitations and uncertainties; therefore, combining them with machine learning algorithms (MLs) could improve predictions and reduce uncertainty. To that end, the DSSAT-CERES-maize model was calibrated in one location and validated in others across Egypt with varying agro-climatic zones. Following that, the dynamic model (CERES-Maize) was used for long-term simulation (1990–2020) of maize grain yield (GY) and evapotranspiration (ET) under a wide range of management and environmental factors. Detailed outputs from three growing seasons of field experiments in Egypt, as well as CERES-maize outputs, were used to train and test six machine learning algorithms (linear regression, ridge regression, lasso regression, K-nearest neighbors, random forest, and XGBoost), resulting in more than 1.5 million simulated yield and evapotranspiration scenarios. Seven warming years (i.e., 1991, 1998, 2002, 2005, 2010, 2013, and 2020) were chosen from a 31-year dataset to test MLs, while the remaining 23 years were used to train the models. The Ensemble model (super learner) and XGBoost outperform other models in predicting GY and ET for maize, as evidenced by R2 values greater than 0.82 and RRMSE less than 9%. The broad range of management practices, when averaged across all locations and 31 years of simulation, not only reduced the hazard impact of environmental factors but also increased GY and reduced ET. Moving beyond prediction and interpreting the outputs from Lasso and XGBoost, and using global and local SHAP values, we found that the most important features for predicting GY and ET are maximum temperatures, minimum temperature, available water content, soil organic carbon, irrigation, cultivars, soil texture, solar radiation, and planting date. Determining the most important features is critical for assisting farmers and agronomists in prioritizing such features over other factors in order to increase yield and resource efficiency values. The combination of CMs and ML algorithms is a powerful tool for predicting yield and water use in arid regions, which are particularly vulnerable to climate change and water scarcity.